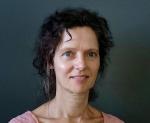
Sara van de Geer
- Born inNetherlands
- Studied inNetherlands
- Lives inConfédération Helvétique
Interview
Sara van de Geer has been Full Professor at ETH Zurich since September 2005. Her main field of research is mathematical statistics, with a special interest in high-dimensional problems. The focus points are empirical processes, curve estimation, machine learning, model selection, and non-and semiparametric statistics. She is a member of very prestigious organizations like the Reseach Council of The Swiss National Science Foundation, Leopoldina German National Academy of Sciences and the International Statistical Institute, and a fellow of the Institute of Mathematical Statistics. She is a correspondent of the Royal Dutch Academy of Sciences and the President of the Bernoulli Society.
“If the statistics are boring, then you’ve got the wrong numbers”, said Edward R. Tufte about your research area. How did you discover the passion for mathematics, and what fascinates you in the random processes?
Indeed, when I started with statistics as a topic for my master thesis, it was not all that popular. How times have changed! Statistics is no longer considered as being boring. On the contrary, it is a very exciting research theme nowadays. Statistics has a substantial role in for example `machine learning’, `data science’, or `information theory’. I discovered statistics as a way to think about `knowledge’. To me, it is a mix of philosophy and mathematics.
Your father was a famous psychologist who studied the psychology of problem-solving. How did he influence your decision to become a mathematician? Could you suggest some approaches that are helpful in the process of formulating and resolving abstract tasks?
There is a Dutch children’s song which I translate:
Three times three is nine
Everybody sings his own song
Three times three is nine
Alice sings her song
And when Alice is finished the above song starts again to have the next person sing his song. When I was about four years of age my father asked: `How much is three times three?’ I immediately answered: `Nine of course!’ This greatly impressed my father. And I could not imagine he didn’t know the song. So my father believed I had mathematical talent and that is how it all started. My father greatly encouraged me to follow the mathematical path.
You ask me about approaches for formulating and resolving abstract tasks. I don’t know really, the best thing to do is perhaps to take a walk and think about it. And then do something else and try not to think about it all the time. The statistical approach to answering some question is to some extent the empirical method and based on the idea of following a pre-specified protocol. But admittedly, this is not possible for human beings and maybe that is our strength. When evaluating data and drawing conclusions from them, one should probably follow the scientific method as good as one can, taking reproducibility and replicability into account. But it is human’s nature if in the process one changes one’s mind (after looking at the data). This may lead to so-called false positives (research results that do not show up in new studies) but on the other hand, it does help science to progress.
Your contribution to the area of empirical processes allowed a break-through in the theory and the applications of penalization methods. The understanding of high–dimensional data originating in genomics or medical imaging could not be conceived without it. What do you think is the biggest progress made in statistics in the last half century and which are the fundamental problems that you consider of large interest in the today’s statistical research?
Thank you! Statistics is undergoing great progress, inspired by the new data structures. I find the concept of sparseness when dealing with high-dimensional data very appealing. Believing in sparseness is like saying: it looks all pretty complicated but behind it, all are some simple mechanisms. So there is nothing new, but the current modelling based on some underlying sparseness idea is quite successful. To me, it provides a handle to understand today’s complex algorithms and also to understand their limitations. A fundamental problem that is also not new but appears in a new dress is that procedures are being developed that on the one hand are shown to be theoretically optimal (in some sense) but on the other hand, one can also prove that it is impossible to know their accuracy. Thus, we have to reformulate our aims when estimating the accuracy of complicated adaptive procedures. In this light, there are current new approaches using conditional inference. This is all very intriguing to me. With the new data, say shapes or graphs or networks, the computational aspect is also a big issue: what is the value of a statistical optimal procedure if it is NP-hard to compute? Conversely, why strive for small computational error below the statistical error? In other words, statistics and optimization theory go hand in hand these days, generating lots of new mathematical theory and insights.
Teaching mathematics, especially to non-mathematicians, can be a challenge. What is your strategy to catch and keep the attention of your audience?
The way I myself learn something is often chaotic. I find it difficult to take the linear way. Nevertheless, to go through the new material in class, a certain straight line is almost indispensable. Yet, for mathematical statistics, one needs many other branches of mathematics: measure theory, analysis, optimization, probability theory and sometimes even differential geometry. So a bit of hand-waving is unavoidable and there goes the linear path! I tell the students that statistics is not a closed set and that’s what makes it a nice challenge. Many students like that, but of course it is a bit of a problem when it comes to preparing for the exam. Therefore I also try as much as possible to offer them a closed set of things to know for the exam.
You became a famous mathematician at a time when concepts like work-life balance were still unknown. Could you tell our readers what is the secret of your personal and professional success?
I have faced some difficulties being a woman in the world of mathematics. When my son was born I got a reprimand because my publication list was considered as too slim. It bothered me quite a bit, but I told myself that I had been very productive in another sense! There is no secret of course. There were many hurdles to take and all I did was just continuing the work. I did not have any special ambition, it was just a job, and a nice one too. For example, I did not really want to do a PhD. I quit a research project because I was not really convinced of the models used. People do not seem to understand such moves. During the years, I did develop a certain ambition, that’s true. Now, I am almost swallowed by the work. But I take my time for doing things in the house. Following the example of my grandmother, I always wear a little apron when I am at home.
What is your opinion about the special mentoring programs in mathematics? What should it be the role of a PhD advisor or of the mentor for a postdoc student?
As a mentor, I so far only have discussed their study programs with the students. And so far they have shown to be very independent and motivated by their choices. There was not much for me to do. What in general the role of PhD or Postdoc advisor should depend, in the situation, I would say. As for PhD students, I am the more experienced one, so my role is in part to help them find feasible research directions, to help avoid dead ends. I tell them about conferences and workshops that they might want to attend, things like that. For the rest, we are scientific collaborators. Mentoring a Postdoc means to me help him or her set up an own research line. It implies among other things that I am most often not a co-author of their papers. Once Postdocs and PhD’s start writing papers with each other I see my mission as being successful.
Do you consider necessary to organize special programs like Girl’s Day promoting science for girls in schools? What do you think it can still be done to support woman careers in mathematics?
There I too have many questions. Do we have such programs to improve science or to improve women’s lives? Are the goals that men set themselves comparable as those of women? Why are more women in statistics than in, say, pure mathematics? (I do not have the exact statistics.) I have experienced much support during my career. For example, I felt people appreciated me as being one of the few female students. Then as time went by it sometimes looked grimmer. And then at ETH, I found again a very welcoming research environment. In my view the best support is allowing people to have a life besides the job.